Full title: A Comparative Evaluation of Probabilistic and Deep Learning Approaches for Vehicular Trajectory Prediction
This work compares two innovative methodologies to predict future locations of vehicles when their current and previous locations are known. The two methodologies are based on a Bayesian network and a deep learning approach based on recurrent neural networks (RNNs).
Several experimental results indicate that the prediction accuracy is improved as more information about prior vehicle mobility is available. The Bayesian method is advantageous because the statistical inference can be updated in real-time as more trajectory data is known. On the contrary, the RNN-based method requires a time-consuming learning task every time new data is added to the inference dataset.
The results show that the computational cost to predict the next position a vehicle will move to can be substantially reduced when the Bayesian network is adopted. But when the quantity of prior data used in the prediction increases, the computational time required by the RNN-based method can be two orders of magnitude lower, showing that the RNN method is advantageous in both accuracy and computational time.
Both methods achieve a next position successful prediction rate higher than 90%, confirming the applicability and validity of the proposed methods.
Full Article: IEEE Open Journal of Vehicular Technology, January 2021
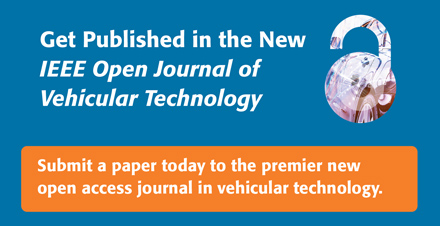
|