Full title—Bridging the Gap Between Point Cloud Registration and Connected Vehicles
Connected vehicles can benefit from sharing and merging their observations, both to develop a more complete understanding of the traffic scene, and also track traffic participants behind obstructions.
Although vehicle-to-vehicle (V2V) communications provide a channel for point cloud data sharing, it is challenging to align point clouds from two vehicles with state-of-the-art techniques due to localization errors, visual obstructions, and differences in perspective.
Therefore, we propose a two-phase point cloud registration mechanism to fuse point clouds which focuses on key objects in the scene where the point clouds are most similar, then infers the transformation from them.
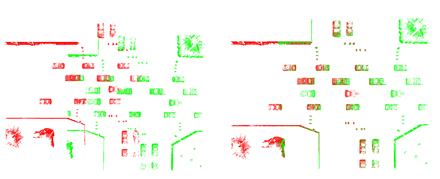
Improved alignment can be seen using the new techniques (right) compared to the current state of the art (left)
Our system first identifies co-visible objects between vehicle views based on hyper-graph matching using multiple similarity metrics, and then refines the overlap region between co-visible objects across the views for point cloud registration.
The system is evaluated based on both experimental and simulation data, which shows tremendous performance improvement when combing with state-of-art baselines.
Full Article: IEEE Open Journal of Vehicular Technology, Early Access
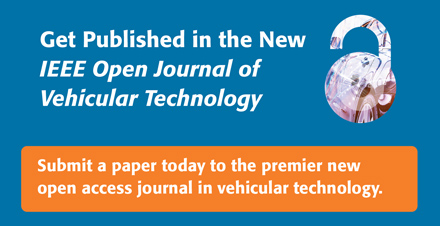
|