Full title: Distributed Estimation Framework for Beyond 5G Intelligent Vehicular Networks
In this paper, we first provide an overview of existing research on IVNs for beyond 5G (B5G) communications, while emphasizing requirements and technical approaches. To fully unleash the potential of vehicular intelligence, smart vehicles should acquire the values of some important variables of interest, e.g. traffic volume in the network.
Thus, we introduce a generalized framework that formulates the acquisition of desired variables as a joint estimation and detection problem. Our framework adopts factor graph to solve problems in IVNs. This is done by collecting the observations from vehicles at road side units (RSUs) for inferring such variables and sending them back to vehicles. Nevertheless, this centralized framework critically depends on the functional reliability of the RSUs.
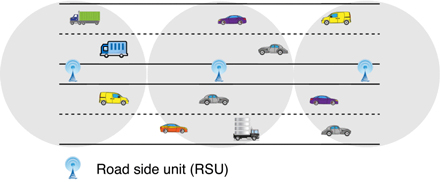
Vehicular network model; coverage of each RSU shown in gray.
To this end, we propose a distributed estimation framework to improve the scalability and robustness, in which vehicles can communicate wirelessly with other vehicles within the communication range. Then, we introduce different consensus operations as a realization of this proposed framework and briefly compare them in terms of implementation feasibility and convergence behavior.
Three approximation schemes are further considered for reducing the required communication signaling overhead. To shed light on the proposed distributed estimation framework, we focus on two cases, i.e., target tracking and network decoding in IVNs. Through simulations, we show that the distributed algorithms can efficiently track the target and decode the broadcasted messages, while achieving the same performance of the centralized scheme.
Full Article: IEEE Open Journal of Vehicular Technology, Volume 1, April 2020
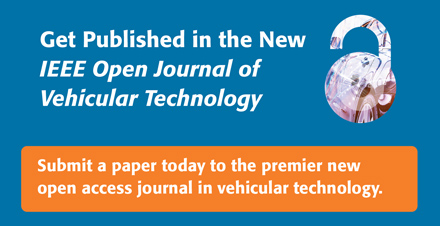
|